Conceived by a global analysis group, the proposed mannequin makes use of the convolutional neural community (CNN) structure U-Net for picture segmentation and the CNN structure InceptionV3-Net for fault classification.
An worldwide analysis staff has developed a brand new PV fault detection technique primarily based on deep studying of aerial pictures.
The proposed technique makes use of convolutional neural community (CNN) structure U-Net for picture segmentation after which makes use of CNN InceptionV3-Net structure for fault classification.
“The presence of mud, snow, chook droppings, and different bodily and electrical issues on the floor of photo voltaic panels can result in power loss,” the lecturers mentioned. “The want for environment friendly monitoring and cleansing protocols in photo voltaic power techniques can’t be emphasised sufficient. Based on that aim, now we have chosen analysis matters to enhance picture processing and classification duties associated to several types of injury to photo voltaic panels.
For the segmentation step of the mannequin, the staff used a publicly accessible annotated database of 4,616 pictures. The aerial footage are divided into six classes: floor cropland, grassland, saline-alkali, shrubwood, water floor, and rooftop. The database is split in a 60%-20%-20% ratio for coaching, validation, and testing, respectively.
A special database with 885 pictures is split by the identical ratio for fault classification. The dataset incorporates six classes of PV points: clear, dusty, chook dropping, electrical injury, bodily injury, and snow lined. Besides the InceptionV3-Net mannequin—which applies the InceptionV3 base with PictureNet weights—the researchers additionally examined different classification fashions for compression. They are Dense-Net, CellNetV3, VGG19, CNN, VGG16, Resnet50 and InceptionV3.
“At first, aerial satellite tv for pc pictures are processed utilizing the U-net structure mannequin with a 256X256X3 enter format, which matches by three levels: enter decoding, encoding integration and decoding, and producing the output,” the group defined.
It additionally highlights that the InceptionV3-Net structure makes use of the InceptionV3 base with PictureNet weights, enhanced with convolutional layers, Squeeze-and-Excitation (SE) blocks, residual connectivity, and world common pooling. The mannequin contains two dense layers with LeakyReLU and batch normalization, ending with a Soft-Max output layer. It additionally makes use of knowledge augmentation strategies equivalent to rotation, shift, shear, zoom, and brightness changes.
“The mannequin was educated utilizing the Adam optimizer with a studying price of 0.0001 and categorical cross-entropy loss,” in addition they mentioned.
Their evaluation confirmed that the proposed InceptionV3-Net achieved a validation accuracy of 98.34% and an F1 rating—which represents the steadiness between precision and recall—of 0.99%. That compares with validation accuracy within the vary of 20.9% – 89.87% and F1 of 0.21-0.92 in competing fashions.
The take a look at outcomes additionally present that the proposed InceptionV3-Net achieves a validation accuracy of 94.35% and an F1 rating of 0.94. This compares with validation accuracy within the vary of 21%-90.19% and F1 of 0.19-0.91 in competing fashions.
“Future work might handle a number of open areas to additional enhance the capabilities of the InceptionV3-Net mannequin,” the researchers concluded. “Applying the mannequin to different renewable power techniques, equivalent to wind generators or hydroelectric vegetation, will take a look at its effectivity. Further optimization of the mannequin for real-time their error could also be outlined as future work to enhance its sensible utility.
The novel strategy is offered in “SPF-Net: Solar panel fault detection utilizing U-Net primarily based deep studying picture classification,” revealed in Energy Reports. The staff included scientists from the American International University-Bangladesh in Bangladesh, King Saud University in Saudi Arabia, and GMR Institute of Technology in India performed the research.
This content material is protected by copyright and is probably not reused. If you wish to cooperate with us and wish to reuse a few of our content material, please contact: [email protected].
Popular content material
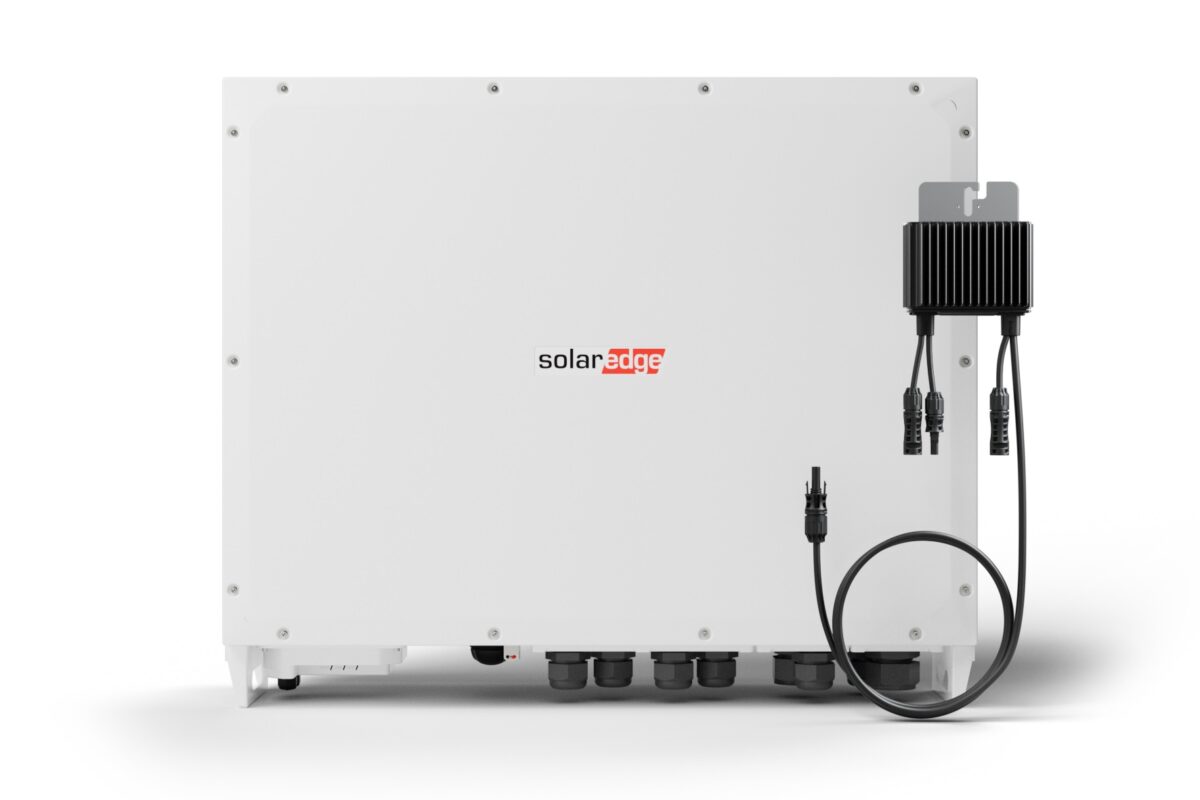