The novel strategy makes use of the YOLOv8 framework, which mixes an consideration mechanism and a transformer mannequin. It was examined on a dataset of 4,500 electroluminescence photographs towards a number of different fashions and its outcomes had been as much as 17.2% extra correct.
Scientists from China have developed a brand new deep studying technique for detecting defects in PV cells. Analyzing electroluminescence (EL) photographs, the novel system makes use of the YOLOv8 convolutional neural community (CNN) structure, which mixes an consideration mechanism and a transformer mannequin.
“To additional enhance the accuracy, I feel we will begin by optimizing the mannequin construction and coaching the loss perform of the mannequin, utilizing extra superior modules or loss capabilities to enhance the accuracy of mannequin whereas making certain detection velocity,” lead creator, Du Lang, mentioned pv journal.
“The EL imaging know-how is essential for picture acquisition, as a result of some anomalies are at all times contained in the PV cells, which can’t be detected by optical or infrared cameras,” mentioned researchers. “Computer vision-based strategies can successfully meet the pressing want for high quality monitoring in varied fields. Automated defect detection is crucial for changing labor-intensive and costly handbook inspections and bettering manufacturing capability.
YOLO is a real-time object detection framework that balances efficiency and effectivity. The newest model improves detection velocity and excessive common accuracy. In their work, the teachers improved the mannequin utilizing the CNN mixed transformer (CCT), which mixes the transformer mannequin to extract international contextual info.
“The transformer mannequin departs from the standard recurrent and convolutional neural community by solely utilizing self-regarding mechanisms to seize the dependencies of the enter sequences,” the scientists defined. “The mechanism of consideration to itself permits the mannequin to guage the significance of various parts of the enter sequence when calculating the illustration of every part.”
In addition to the transformer mannequin, lecturers add the mechanism of polarized self-attention (PSA) to deal with fusion conflicts. They additionally clarify that the strategies are supposed to focus consideration on essential areas of the picture whereas rejecting irrelevant elements referred to as the eye mechanism. “The PSA mechanism introduces the polarization of self-attention mechanisms, permitting the mannequin to concentrate on informational parts whereas selectively suppressing distractions,” they added. “PSA improves the discriminative energy of self-attention mechanisms by assigning polarities to attentional weights, thereby bettering function illustration and process efficiency.”
The novel mannequin was examined on the PVEL-AD dataset, which comprises 4,500 photographs of cells. The cells are annotated in 12 classes, together with crack, finger, black core, thick line, star crack, nook, fragment, scratch, horizontal dislocation, vertical dislocation, printing error, and quick circuit. For reference, seven totally different detection fashions had been additionally run on the identical dataset.
To examine them, the group used the F1 analysis metric, which mixes precision and recall. In addition, it additionally makes use of the imply common precision (mAP) of intersection over union (IoU) 50 (mAP50) and mAP50:95. The first measures the mAP of the mannequin at an IoU threshold of fifty%; the latter evaluates it at a number of IoU thresholds from 50% to 95%, in jumps of 5%.
Their evaluation reveals that Compared with YOLOv3-tiny/YOLOv5u-s/YOLOv6u/YOLOv8, the proposed technique improves F1 rating by 8.4%/2.4%/6.5%/8.7%, will increase mAP50 by 15.5%/ 17.2%/20%/ 16.9% and mAP50:95 of twenty-two.1%/8.4%/9.7%/8.6%.
“Based on the experimental outcomes, our proposed technique achieved a mAP50 of 77.9%, surpassing YOLOv8, which achieved 61.0% mAP50, displaying superior efficiency in F1 rating, mAP50 , and mAP50: 95 in comparison with different YOLO detectors,” the scientists concluded. “Although the frames per second (FPS) of the proposed technique is barely diminished in comparison with the baseline, it meets the real-time calls for of manufacturing traces and achieves a big efficiency enchancment .”
The new mannequin is introduced in “A PV cell defect detector mixed with a transformer and a spotlight mechanism,” revealed in Scientific reviews. Scientists from China’s Zhejiang Industry Polytechnic College and Northeastern University carried out the research.
This content material is protected by copyright and will not be reused. If you need to cooperate with us and need to reuse a few of our content material, please contact: [email protected].
Popular content material
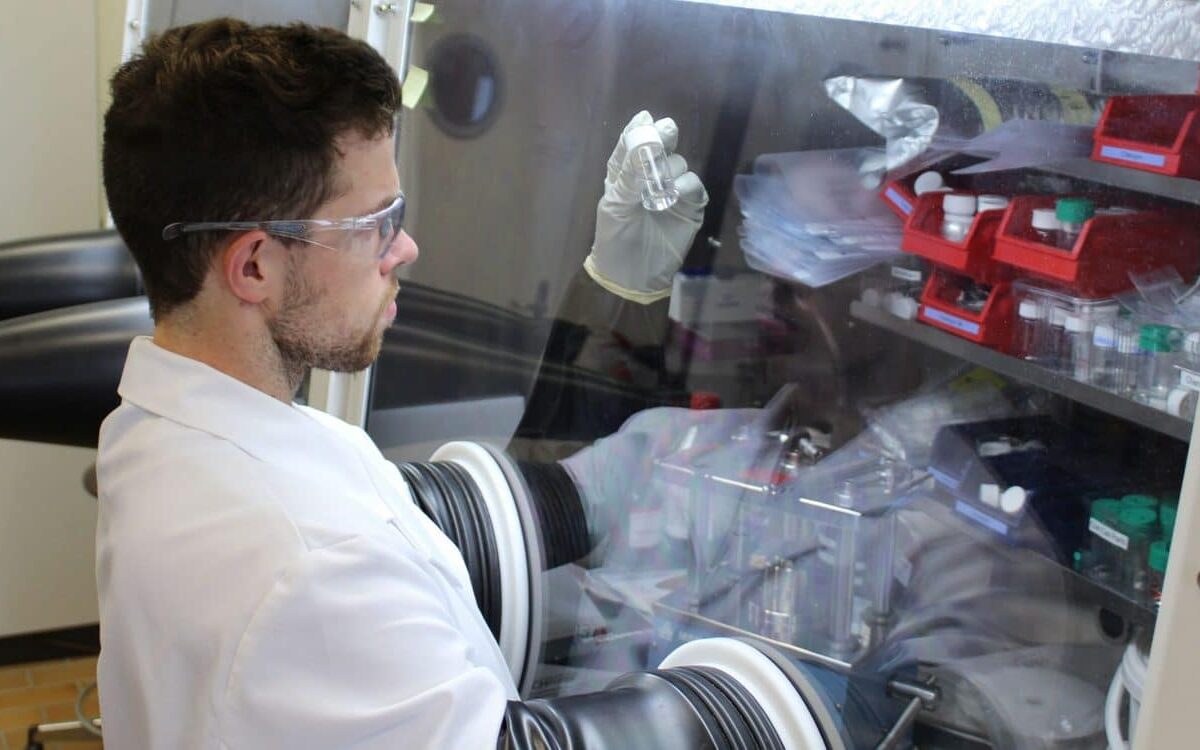