[ad_1]
Iraqi scientists used a k-Nearest Neighbors algorithm to judge the operational standing of PV modules below completely different circumstances, together with partial shading, open circuit, and quick circuit eventualities. They discovered that the general efficiency of the mannequin in predicting energy output was “probably the most correct.”
Scientists from the University of Technology – Iraq used the k-Nearest Neighbors (k-NN) machine studying (ML) algorithm to categorise the operational standing of PV panels and predict their voltage, present, and energy output.
“At its core, k-NN works on the precept that comparable objects are in shut proximity. In sensible phrases, it may be understood that the algorithm predicts the label of a brand new knowledge level based mostly on the bulk vote or common of its ‘k’ nearest neighbors within the function area,” the scientists defined. “In classification duties, k-NN is used to categorize new observations into predefined courses. based mostly on the most typical class of its nearest neighbors.”
The analysis workforce skilled the algorithm on a dataset generated by means of Python and LTspice XVII software program, utilizing a 2-diode-based equal PV module modeling approach. It produced nearly seven million circumstances displaying a large spectrum of circumstances, together with parameters resembling photo voltaic irradiation ranges, PV panel configurations, fault circumstances, partial shading circumstances, and temperature variations.
Half of the dataset is used for coaching, whereas the opposite half is used for testing. For classification functions, scientists use the accuracy and F1 metrics. For energy output forecast, they used two evaluation metrics in regression evaluation – root imply sq. error (RMSE) and coefficient of willpower (R2).
“The classification accuracy for the primary categorization was famous to be 99.2% whereas it barely dropped to 98.9% when classifying the subcategories,” the scientists mentioned. The k-NN mannequin additionally recorded an RMSE rating of 0.036 for voltage prediction and an R2 of 1.00. As for the present, it exhibits an RMSE of 1.813 and an R2 of 0.878, whereas, by way of energy, the evaluation exhibits an RMSE of 0.371 and an R2 of 0.994.
“Although the prediction of the present exhibits some challenges, the general efficiency of the ability output prediction mannequin could be very correct, additional validating the potential of ML strategies in bettering the effectivity and reliability of photo voltaic power programs,” the workforce concluded. “Through this investigation, the examine not solely confirmed the viability of simulation-based knowledge in pre-experimental assessments but additionally highlighted the vital function of ML, particularly the k-NN algorithm, within the growth of photo voltaic power analysis and software.”
Their findings are introduced within the examine “Evaluation {of electrical} energy yield of photovoltaic photo voltaic cells with k-Nearest neighbors: A machine studying statistical evaluation strategy,” revealed in e-Prime – Advances in Electrical Engineering, Electronics and Energy.
This content material is protected by copyright and might not be reused. If you wish to cooperate with us and wish to reuse a few of our content material, please contact: [email protected].
Popular content material
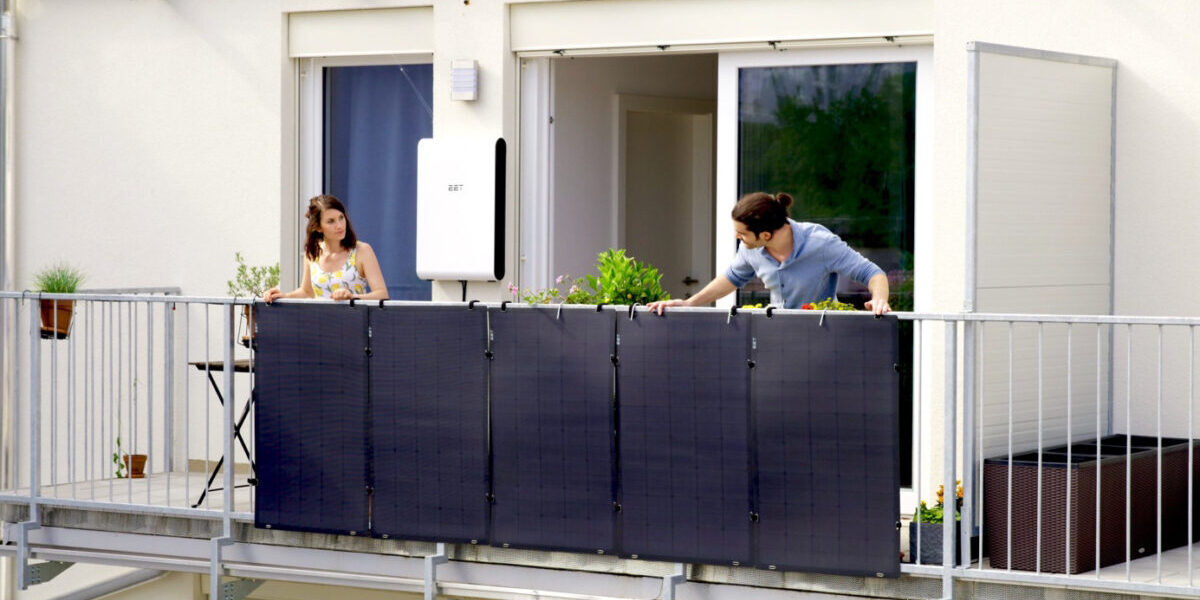
[ad_2]
Source link