Using a surrogate mannequin for finite factor evaluation, scientists designed an aluminum body for bifacial modules that reportedly reduces deflection and manufacturing price, with out including weight. Deflection can result in cracking or delamination of the ribbons inside the module, leading to a rise in broken cells.
Scientists from the Korea Institute of Industrial Technology in South Korea have developed a deep studying surrogate mannequin for optimizing module aluminum (Al) frames utilized in glass-glass bifacial photovoltaic panels.
Scientists say that as a result of rising measurement and weight of PV modules, Al frames endure from the next threat of deflection, which is the diploma to which part of an extended structural parts are laterally deformed below a load.
“If the module undergoes deformation as a result of load, this may increasingly result in cracking or delamination of the ribbons inside the module, ensuing within the proliferation of lifeless cells,” defined the group. “Bifacial modules are comparatively heavier in comparison with monofacial modules. Therefore, when subjected to extra floor space, there’s a greater chance of the body altering because of its personal weight, which results in the potential module deflection.
To design their optimization mannequin, the lecturers first recognized 5 design elements for the body, together with ridges, grooves, and a hole part. Setting three millimeter ranges for every issue, the group carried out 243 experiments to determine the deflection by way of finite factor evaluation (FEA). FEA is a numerical methodology used to unravel issues in engineering and mathematical physics.
However, as FEA requires important computational sources, the group developed a alternative module. “Deep neural community (DNN)-based surrogate modeling includes discovering an approximate perform that minimizes the loss perform for the given knowledge,” they defined. “We apply Bayesian optimization, which takes hyperparameters that decrease the loss perform.”
Bayesian optimization is a sequential design technique for international optimization of black-box features that don’t assume any practical kinds. This is especially efficient for situations the place sampling is dear, and the target perform is unknown however might be sampled.
Image: Korea Institute of Industrial Technology, Scientific Reports, CC BY 4.0
Using the outcomes of 243 experiments and artificially augmenting them, the group then educated and examined the novel mannequin with a ratio of 9:1 every. Compared to the true FEA, the FE surrogate mannequin exhibits excessive accuracy in imply absolute share error (MAPE) and coefficient of dedication (R2) values for deflection and weight which is a median of 0.0017, 0.9972 for within the coaching set, and 0.0020, 0.9962 for the take a look at set, respectively.
After the optimistic outcomes, the lecturers moved to optimize the body of a big measurement 585 W bifacial PV module with 78 M10 cells. It has a business AL body weighing 3.2 kg. This body causes a deflection of about 12.3 mm with a displacement of roughly 2.8 mm in the wrong way.
Popular content material
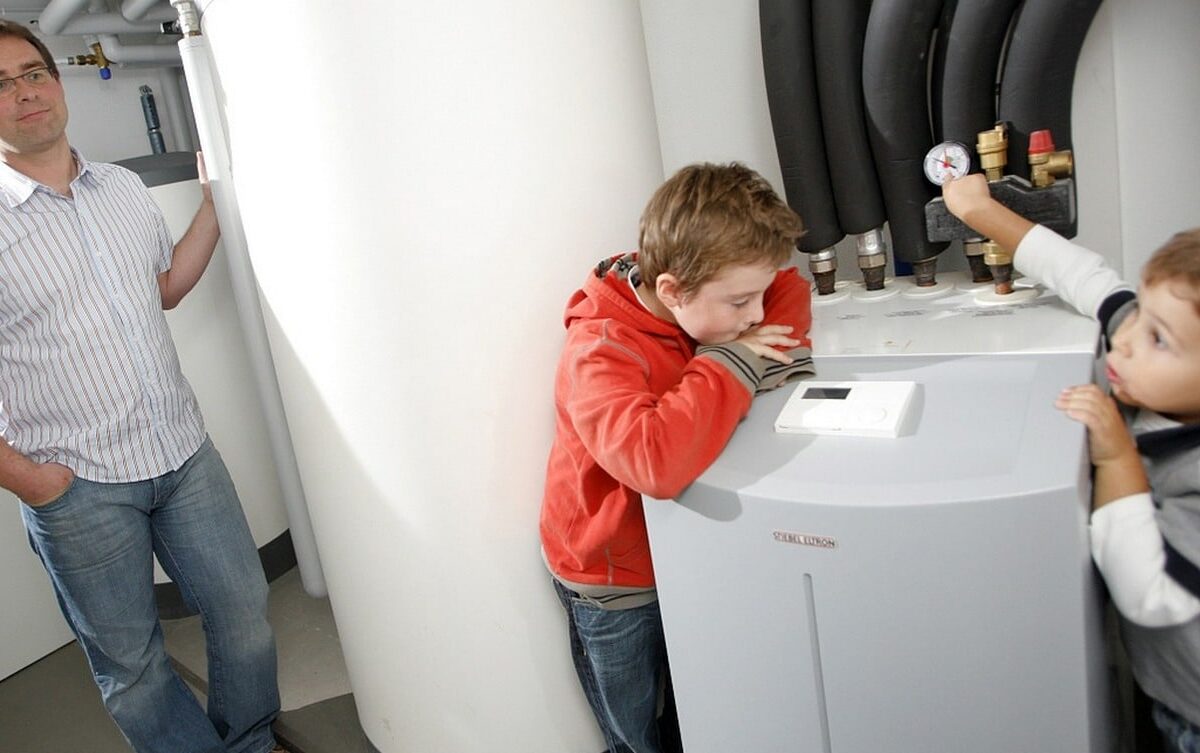
The purpose of optimizing the novel mannequin is to design a body that minimizes deflection with the bottom attainable weight, which signifies that making a body thicker can considerably cut back deflection however improve additionally the price of manufacturing. “The values of the issue that reduces the deflection and weight are discovered to be a = 1.5176 mm, b = 13.7105 mm, c = 1.5012 mm, d = 2.9898mm, e = 4.3123. At this level, the deflection is 11.1 mm, and the load is 3.6 kg,” mentioned the scientists.
Image: Korea Institute of Industrial Technology, Scientific Reports, CC BY 4.0
The outcomes have been achieved after the brand new optimization mannequin checked out one million datasets. “Creating 1 million datasets takes 0.957 seconds (s), acquiring the expected deflection and weight values for these datasets takes roughly 72.014 s, and discovering probably the most good values from these predictions took about 0.264 s. In distinction, utilizing conventional FEA to acquire deflection and weight for a case took about 4,800 s,” they mentioned.
Using their novel methodology, they reportedly diminished deflection by round 9.6% whereas rising body weight by round 12.8%.
Their findings are introduced within the examine “Design optimization of enormous bifacial photovoltaic module body utilizing deep studying surrogate mannequin,” revealed in Scientific studies.
This content material is protected by copyright and is probably not reused. If you wish to cooperate with us and wish to reuse a few of our content material, please contact: [email protected].